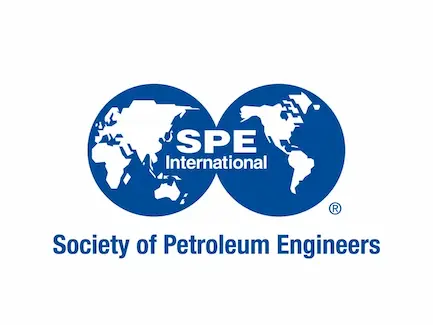
Geological Neural Network Methodology for History Match
Full history match models in subsurface systems are challenging due to the large number of reservoir simulations required, and the need to preserve geological realism in matched models.
Full history match models in subsurface systems are challenging due to the large number of reservoir simulations required, and the need to preserve geological realism in matched models.
Physics-informed neural networks have enabled significant improvements in modelling physical processes described by partial differential equations (PDEs) and are in principle capable of modeling a large variety of differential equations.
Generative Adversarial Networks have shown impressive results for image generation. However, GANs face challenges in generating contents with certain types of constraints, such as game levels.